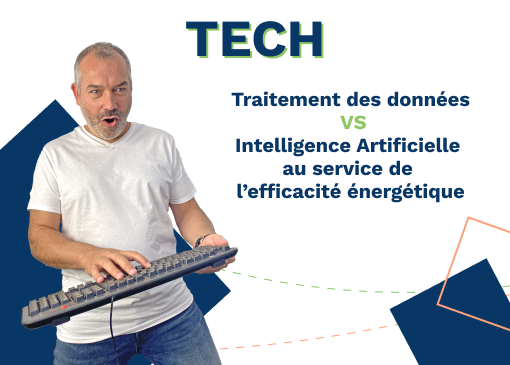
Data Processing vs Artificial Intelligence for Energy Efficiency
Manual data processing and artificial intelligence are often presented as opposites. At Dametis, our experience shows that human experience is still necessary to achieve better results with Artificial Intelligence, particularly in the field of energy efficiency.
Jérémy Barrais, Product Manager at Dametis, talks about the advantages of the Dametis data processing method over conventional big or smart data.
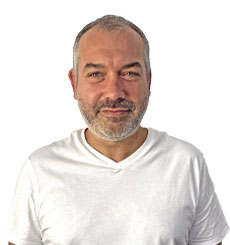
The difference between Big Data, Smart Data and the data models used by Dametis
Big Data: A maximum amount of data is provided to the AI
Due to the heterogeneous nature of Big Data, correlations can be found at random. The large number of parameters involved affects the accuracy of the various correlations. Worse still, some may be completely meaningless.
Correlations of this kind have already been carried out. For example, an AI has associated the number of ice-cream sales and drownings, even though the two have no causal link. They just have the same cause, the increase in beach visits.
Another example: it has been observed that the number of flushes in one southern US state was proportional to the number of divorces in the opposite state. But there’s no link between the two.
Smart Data: Data is selected by theme, to be limited to a functional context.
With Smart Data, data is grouped by theme, limiting AI to using data from relevant functional scopes. In this way, we ensure that reconciliations are made with data that may have a causal relationship between them. The challenge is not to delimit the perimeter too much, so as not to exclude upstream factors that may ultimately have an influence.
Business expertise remains fundamental at this stage. Our Dametis specialists carry out on-site measurement plans, a fundamental element of smart data, enabling the selection of the most significant and powerful data.
Dametis model: data is contextualized before analysis by AI.
Thanks to their in-depth expertise, Dametis specialists know which perimeters of an industry can be correlated, and which plant data the artificial intelligence needs to examine to propose optimizations, detect anomalies and drifts.
The block diagram, a support for contextualizing data
Dametis will then give context to the various data to enrich the AI’s analysis.
To do this, Dametis will first define the physical relationships between the data and build a “schema”.
In addition to this mesh, the data will be fed into “blocks” representing the different installations of a plant (itself a block in its own right, made up of “child” blocks, which is the basis of our “Lego theory”).
These blocks are parameterized by our experts to integrate all business logic. This mesh and these blocks represent our “block diagram” concept.
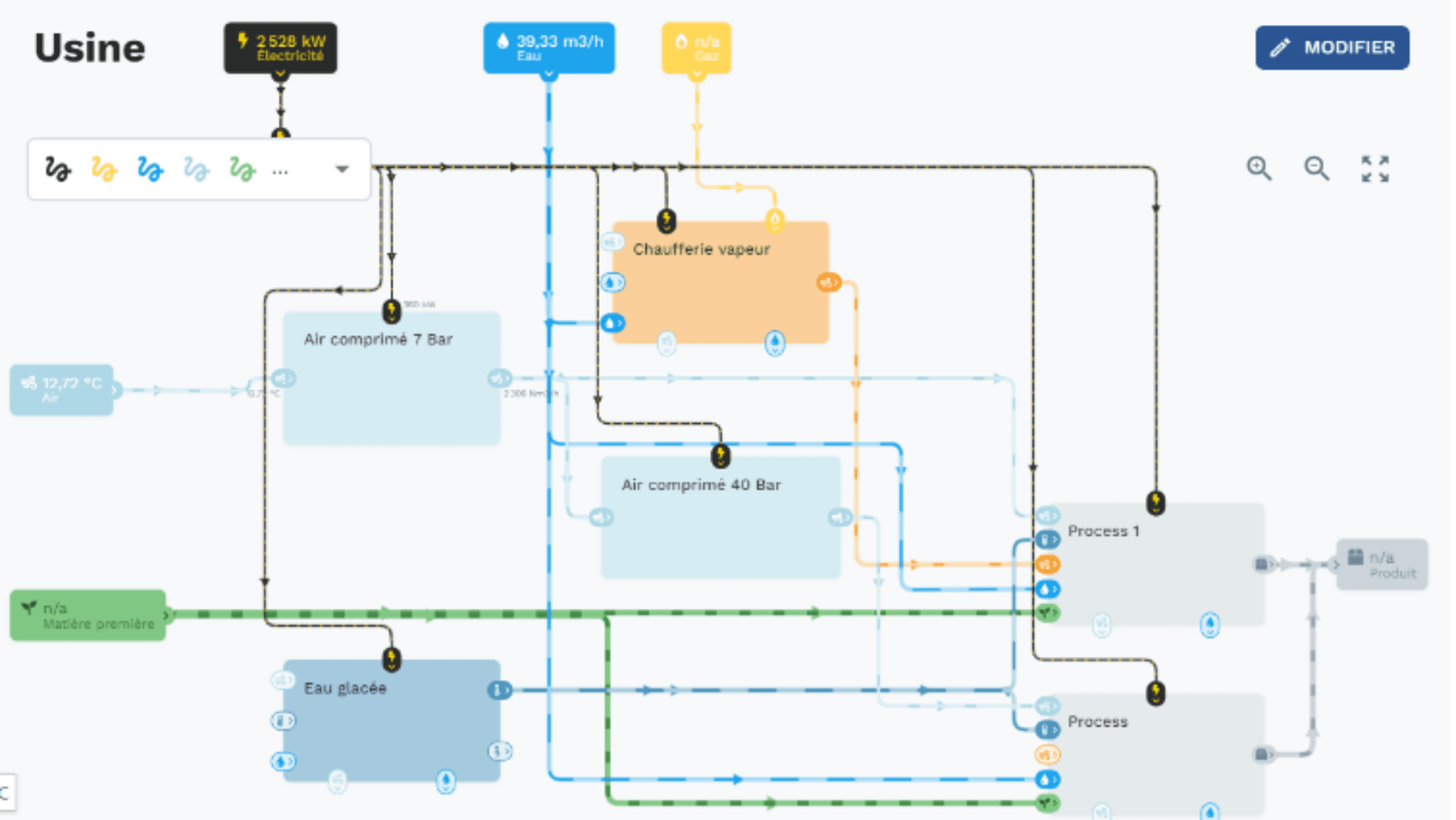
The block diagram, a concept for refining predictions
Once constructed, the block diagram makes it easy to map a plant, utility, process or piece of equipment.
For example, the temperatures of a fluid at the various stages of its circulation loop (central outlet, exchanger 1, exchanger 2 inlet, central return, etc.) will no longer be evaluated independently, but in relation to each other. For a compressed air system, compressor M/A, power consumption, flow rate and central outlet pressure are all linked together to provide a “link” between the data.
By associating them with a block diagram representing the plant, additional contextual information is added to the data, which is then fed with knowledge, known as metadata.
The operating conditions of each block will also be documented to provide even more information, such as operational instructions.
This contextualization in the form of a block diagram enriches the collected data, thereby refining the modeling and predictions.
Imagine specialists working 24/7 on optimizing your industrial sites. That’s what MyDametis offers you, the only platform designed 100% by environmental performance experts.
A model with multiple benefits
Jeremy Barrais reminds us that, although Dametis’ initial actions focus on energy, today, the examined areas go further, including material losses and optimizing in-place washing times (NEP).
Once the model is configured by our experts, AI will use all these elements to model your entire installation, from the smallest component to the largest assembly.
Numerous benefits stem from this.
Real-time
You can view your data and modeled data in real-time. You can thus see those that are defective or drifting. MyDametis’ graphical analysis module optimization also allows you to visualize data over different aggregation periods, ensuring you always have the most appropriate macro view for the observed phenomenon.
You can easily switch to “second” views for temporal analysis of operation, “hourly” views for consumption profiling, or “daily” or “monthly” views for consumption tracking and matching them to your bills.
Drift analysis
This real-time capability allows you to have complete control over your installations. Nothing will be left to chance as even the slightest drift is visible on your platform.
Automatic notifications
You receive automatic notifications as soon as a data or analysis is suspected. For example, in areas where material loss volume suddenly becomes significant. Also, you receive alerts when there is a technical failure.